The process of collecting, arranging, and selecting data from various sources to feed AI algorithms is known as AI data collection. AI systems use data to spot patterns and carry out operations previously limited to humans.
However, applications using artificial intelligence need high-quality data to function fully. In other instances, though, businesses must gather more information to guarantee a robust data pipeline that will support their AI implementations for assessment, testing, or training.
Large-scale data collection is complex, especially concerning current regulations and privacy laws. Furthermore, it takes more work to complete a large-scale or complex data collection project when data is needed from locations worldwide.
For these reasons, collaborating with an AI data collection service provider can significantly speed up the development of trustworthy data pipelines and assist companies in making the smoother and faster transition from pilot to production.
Types of AI Data Collection
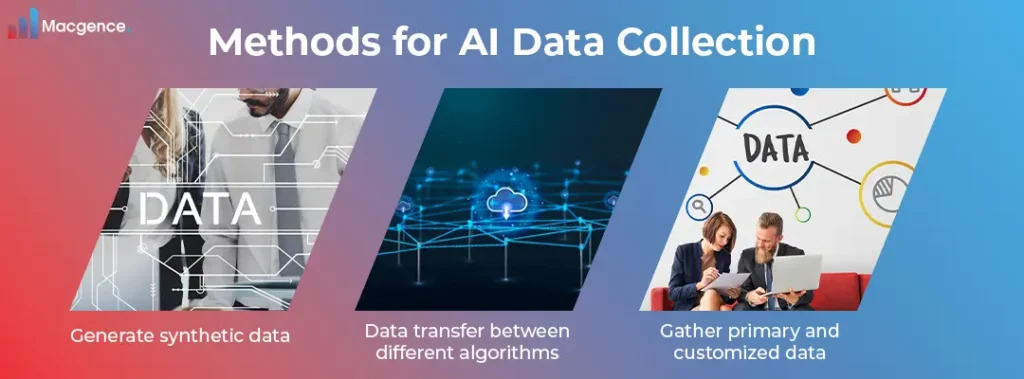
Numerous data collection forms are available for AI/ML models, and each type has a distinct set of uses. Furthermore, knowing the particulars of the data collection process can impact the method selected for a given AI/ML model. Let’s examine the different approaches to data collection that the AI/ML models employ.
Image Data Collection
Big and small data collection is necessary for training AI and ML algorithms. For AI models to advance, image datasets are essential. With their wide range of visual data, they help with efficient pattern learning and recognition. This supports training and testing, improving the models’ overall performance. These datasets support AI models’ recognition and comprehension of intricate visual concepts, enhancing accuracy and dependability across tasks such as object detection, image classification, etc. It features pictures of cars, streets, people, and fruits, along with many other images.
Video Data Collection
The process of assembling a specific kind of video dataset for AI/ML model training is known as video data collection. Video datasets are gathered to help artificial intelligence models comprehend and learn about their surroundings. This allows AI systems to identify objects in moving images. These datasets include CCTV footage, traffic videos, logistics videos, retail videos (supermarkets), and recordings of human activity. For the development and training of algorithms, a large, varied, and easily accessible high-quality video dataset is necessary.
Audio Data Collection
Using high-quality audio datasets, your machine learning and artificial intelligence (AI) solutions will be accurate. Speech data collection is required to improve voice assistants’, speech-to-text conversions, and other voice-enabled applications’ accuracy and efficiency. Audio data collection is the process of gathering and analyzing audio and speech data methodically. Gathering audio recordings from various sources, including calls, call centers, consultations, baby sounds, accents, etc.
Text Data Collection
AI applications must have a wide range and high-quality training datasets to succeed. Text datasets for NLP play a critical role in teaching AI systems how to comprehend and analyze natural language. Machine learning models perform better when text data is used, with a focus on precise and innovative AI. Prescriptions, handwritten notes, PDFs, clinical records, bank documents, and other text datasets must all be gathered and categorized.
3D Point Cloud Data
Enhancing the automotive industry requires accurate 3D point cloud data. LiDAR data is necessary for precise detection using lidar sensors with 3D boxes for AI systems to train autonomous cars efficiently. Only with the best quality high-resolution 3D data can lidar sensor performance in autonomous systems be improved.
Methods for AI Data Collection
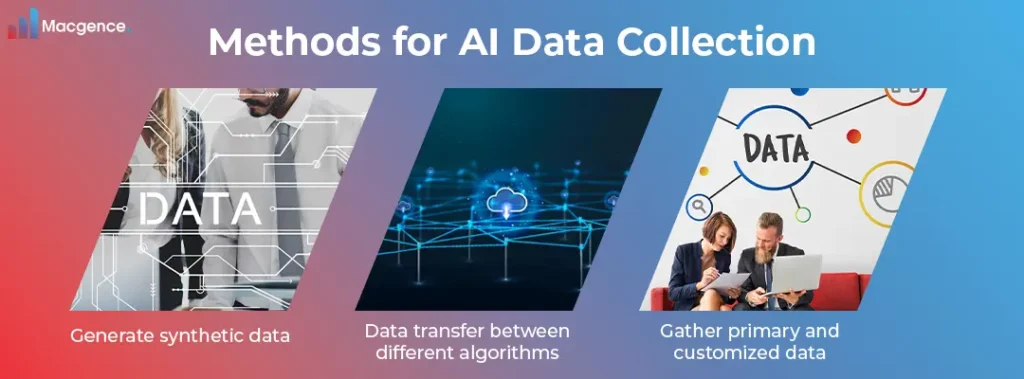
Generate synthetic data
Companies can use a synthetic dataset based on an original dataset and then expand upon it instead of gathering data from the real world. Synthetic datasets aim to replicate the original’s features while eliminating any inconsistencies (although the absence of probable outliers could result in datasets that only partially capture the essence of the issue you’re attempting to solve). Synthetic datasets could be a great way to advance your experience if your company is in financial services, telco, healthcare/pharma, or other industries with strict security, privacy, and retention policies.
Data transfer between different algorithms
Alternatively referred to as transfer learning, this data collection technique uses an existing algorithm to train a new algorithm. This approach offers definite advantages in terms of cost and time savings; however, it is only effective when moving from a general algorithm or operational context to a more focused one. Transfer learning is frequently applied in natural language processing, which uses written text, and predictive modeling, which uses still or video images. For example, transfer learning is used by many photo management apps to create filters for friends and family, making it easy to find every photo in which they are featured.
Gather primary and customized data.
The best starting point for training a machine learning algorithm is gathering raw data from the field that satisfies your requirements.
Get Started with Macgence:
At Macgence, we comprehend. We know how essential AI data collection services are to the success of companies. Imagine having a lot of data. However, where do you even begin? Here’s where we get involved. We have years of experience and cutting-edge technology.
We’re on it from the time we begin collecting until the end of the analysis. We are discussing state-of-the-art AI that ensures every piece of data is examined, analyzed, and transformed into insights that can be implemented.
When Macgence is on your side, you get more than just a service provider.
Conclusion:
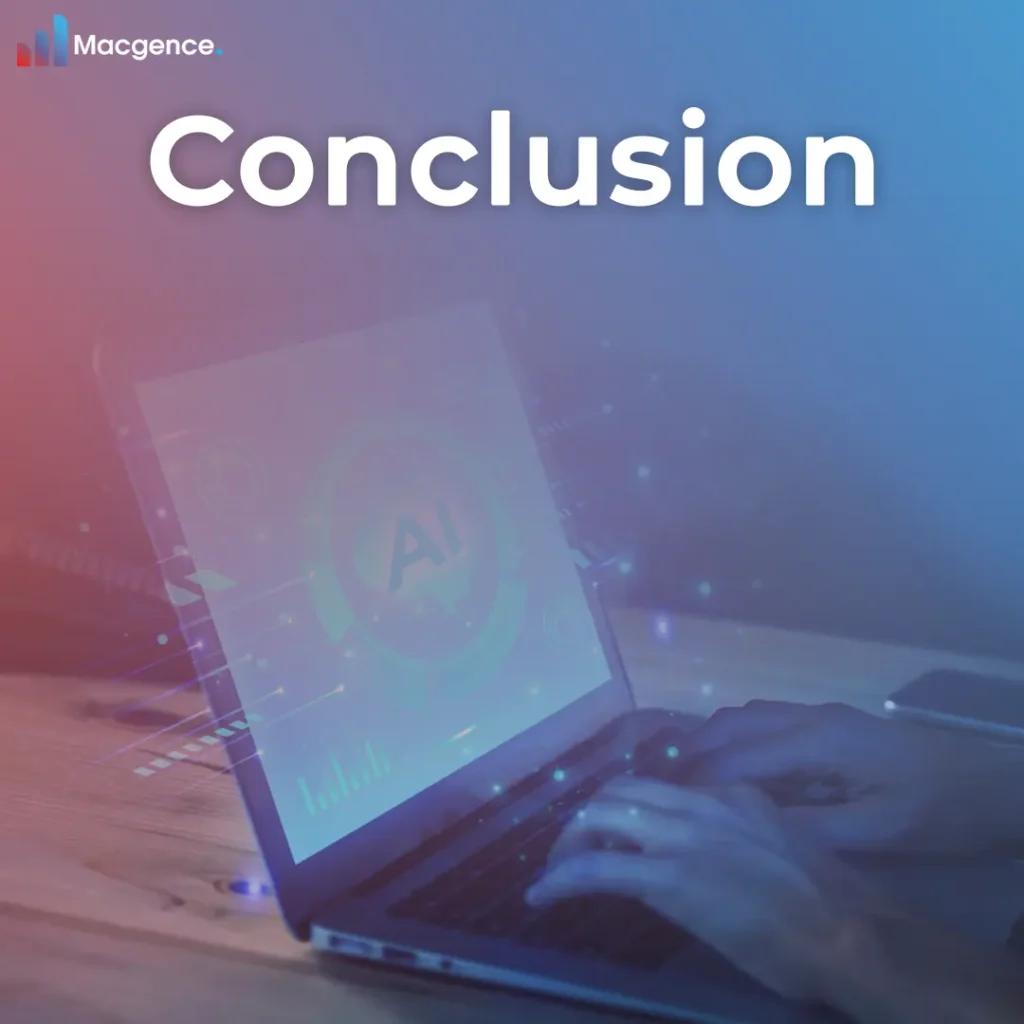
Finding outside training data is a reasonable option regardless of your company’s level of AI/ML maturity, and these strategies and methods for gathering data can help you grow your AI/ML training datasets to suit your needs. However, it remains imperative that both internal and external training data sources are integrated into a comprehensive strategy.
By developing this plan, you will be able to see your data more clearly, identify any gaps that could negatively impact your company, and determine the best ways to gather and handle data to maintain the momentum of your AI/ML development.
FAQs:
Ans: – The process of compiling and evaluating massive amounts of data with artificial intelligence algorithms is known as artificial intelligence data collection.
Ans: – Data quality, bias, and privacy are challenges in collecting AI data. Nevertheless, these difficulties can be overcome by carefully organizing and implementing best practices.
Ans: – Macgence offers specialized solutions to problems with their years of experience and cutting-edge technology in AI data collection.