Artificial Intelligence (AI) has rapidly transformed industries, enabling smarter decisions, streamlined operations, and innovative new products. But what sets apart truly intelligent AI agents from mediocre ones? The answer often lies in the data they’re trained on—and not just any data, but Domain-Specific Data for AI Agents.
If you’re a data analyst, AI developer, or tech enthusiast, understanding how Domain-Specific Data for AI Agents empowers AI to excel can elevate your projects and improve your outcomes. This blog explores why this type of data is critical, how to gather it, the challenges involved, and the exciting future it holds for AI development.
Understanding Domain-Specific Data for AI Agents
What is Domain-Specific Data?
Domain-specific data relates to a specific field, industry, or context that is exceptionally relevant for that area. Unlike general data that serves a wider purpose, domain-specific data is designed to fulfill niche requirements.
For example:
- Healthcare AI makes use of life history information, diagnostic images and other particular medical treatments and their outcomes.
- Finance focused AI utilizes stock value, market movement, and trading volume information.
- Retail AI utilizes customer behavior, inventory status, and product suggestions.
How is Domain-Specific Data Different from General Data?
While general data trains AI systems for broader functions (e.g., natural language processing or general image recognition), Domain-Specific Data for AI Agents refines models for specialized use cases. The difference is in precision:
- General Data provides AI with a baseline understanding.
- Domain-Specific Data for AI Agents fine-tunes that baseline into mastery within a given domain.
For instance, while a general speech recognition AI might struggle to understand medical jargon like “tachycardia” or “angioplasty,” an AI trained specifically for healthcare thrives thanks to its high-quality, specialized datasets.
Collecting and Preparing Domain-Specific Data for AI Agents
Strategies for Collecting Domain-Specific Data
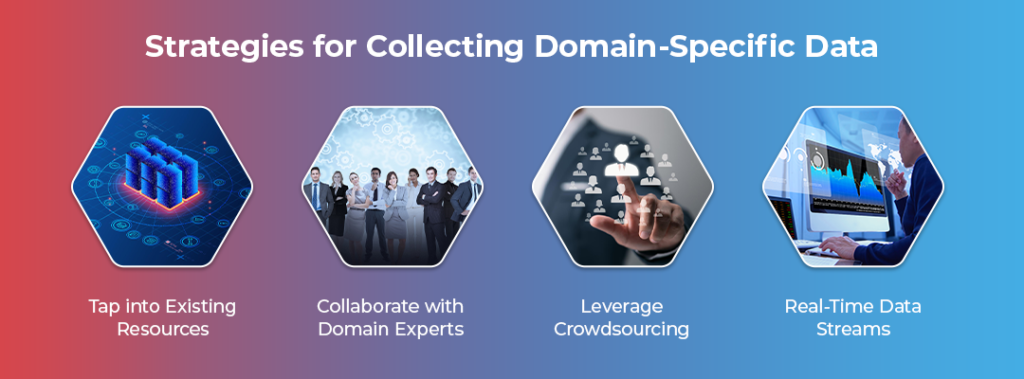
- Tap into Existing Resources: – Many industries already generate massive amounts of domain-specific data. Publicly available datasets, industry reports, and proprietary data offer a wealth of information.
- Collaborate with Domain Experts: – Partnering with experts ensures access to accurate and valuable datasets. For example, collaborating with doctors for medical AI or supply chain managers for logistics-focused AI yields insightful data.
- Leverage Crowdsourcing: – Platforms like Amazon Mechanical Turk help gather data across diverse and niche contexts, building robust Domain-Specific Data for AI Agents.
- Real-Time Data Streams: – Use modern tools to capture real-time data, such as IoT telemetry streams or live finance market feeds, to create dynamic datasets.
Tools and Technologies for Data Preparation
After collecting the data, ensuring it is clean, accurate, and ready for training is critical for AI development. Here’s how:
- Data Cleaning Tools: Tools like OpenRefine or Python libraries (e.g., Pandas) streamline error removal.
- Data Annotation Platforms: Solutions such as Labelbox specialize in tagging domain-specific data to bolster its utility for AI/ML models.
- ETL Pipelines: Efficient Extract, Transform, Load workflows preprocess raw data for better AI readiness.
- AI-Driven Preprocessing: AutoML platforms like Google Cloud AutoML optimize preprocessing using machine learning.
The Role of Domain-Specific Data in AI Development
AI Accuracy and Performance
Training AI agents with Domain-Specific Data for AI Agents enhances accuracy, aligns AI with industry-specific practices, and improves context comprehension. Language models, for example, benefit from specialized legal datasets to interpret contracts and statutes with precision.
Real-World Examples
- Healthcare AI: – IBM Watson Health leverages domain-specific data to deliver accurate diagnostics and treatment plans, making breakthroughs in oncology.
- Retail AI: – Companies like Amazon utilize customer behavior and sales data to power recommendation engines, creating more engaging shopping experiences.
- Self-Driving Cars: – Autonomous vehicle technology relies heavily on specialized datasets, including traffic patterns and weather conditions. Tesla, for instance, analyzes millions of driving hours to refine its AI systems.
Challenges and Solutions in Using Domain-Specific Data for AI Agents
Common Challenges
- Data Scarcity: – Niche industries often face a lack of ready-made datasets, requiring creative and resource-intensive data collection strategies.
- Privacy and Security Concerns: – The healthcare and finance sectors manage sensitive credentials, therefore complying with laws such as HIPAA and GDPR is necessary.
- Data Bias: – Domain-specific datasets sometimes reflect inherent biases, which can negatively impact AI outcomes.
- Complexity of Annotation: – Annotating domain-specific data correctly is resource-intensive and usually requires domain expertise.
Best Practices to Overcome Challenges
- Augment Datasets with synthetic data generation techniques to expand limited data.
- Ensure Privacy Compliance by using tools like federated learning or differential privacy to protect sensitive data.
- Mitigate Bias using bias detection tools like IBM AI Fairness 360 while conducting regular audits.
- Collaborate with Experts to annotate datasets effectively and ensure high-quality results.
Future Trends and Implications
Emerging Technologies & Methodologies
The future of AI lies in enhancing Domain-Specific Data for AI Agents through cutting-edge innovations such as:
- Synthetic Data Generation to simulate cost-effective and diverse datasets.
- Federated Learning to train AI on distributed datasets without compromising privacy.
- Explainable AI, which promotes transparency by making AI systems easier for industry stakeholders to understand.
Industry Impact
- Healthcare will advance personalized treatments with domain-specific datasets.
- Manufacturing will implement predictive maintenance, boosting operational efficiency.
- Finance will refine fraud detection as tailored datasets empower models.
Why Domain-Specific Data for AI Agents is the Future
The future of AI depends on mastering Domain-Specific Data for AI Agents, which empowers systems to perform at their best within specific industries or fields. It improves accuracy, reduces bias, and fosters innovations uniquely suited to niche demands.
Macgence aids businesses by offering industry specific data of exceptional quality for the purpose of creating AI/ML models. We can help maximize the value of your AI, be it building chatbots for customer service, training self-driving cars, or developing healthcare diagnostic systems.
Start building truly intelligent AI agents with Macgence today!
FAQs
Ans: – Domain-specific data tailors AI systems to excel in niche industries or tasks, dramatically improving accuracy and context understanding.
Ans: – Specialized datasets yield maximum benefits for industries such as health care, finance, manufacturing, retail, and logistics.
Ans: – Utilizing public datasets, forming expert partnerships, employing synthetic data techniques, and leveraging annotation platforms are effective strategies.

Macgence is a leading AI training data company at the forefront of providing exceptional human-in-the-loop solutions to make AI better. We specialize in offering fully managed AI/ML data solutions, catering to the evolving needs of businesses across industries. With a strong commitment to responsibility and sincerity, we have established ourselves as a trusted partner for organizations seeking advanced automation solutions.