The importance of data annotation in machine learning for the quickly developing field of road photography cannot be emphasized. The importance of deep learning in machine vision highlights the necessity of properly labeled, high-quality data. This data serves as the foundation for effective road picture collecting, enabling Machine Learning algorithms to precisely decipher complex patterns. complicated approaches such as Semantic Segmentation become essential when road circumstances get more complicated. By breaking up photos into discrete parts, these methods improve the ability of Machine Learning models to analyze and understand photographs of roads. In this post, we examine the key components of premium data annotation services designed specifically for road photography and discuss how they may be used to streamline the data labeling and annotation procedures.
In this article, we’ll discuss the salient characteristics of premium data annotation services, the function of machine learning in road imaging data annotation, obstacles in data annotation, upcoming developments, and the special benefits that Macgence provides in road imaging services, specifically in automated data labeling with machine learning.
Key Features of Top-Tier Data Annotation Services
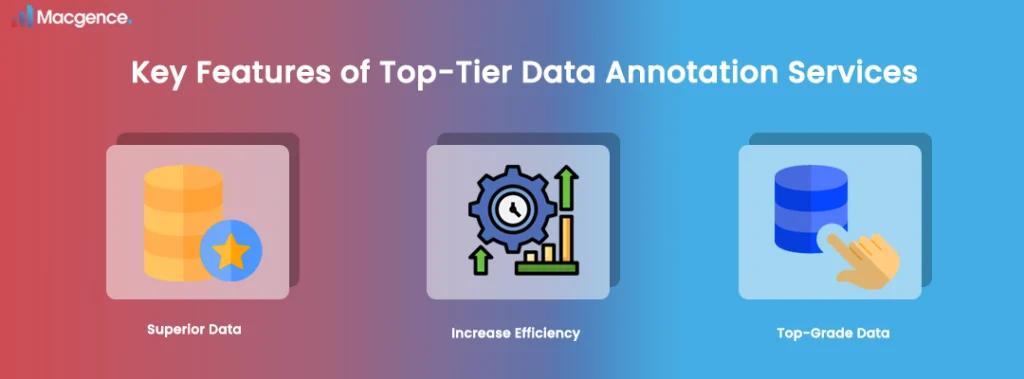
Let’s have a look at the key features of top-tier data annotation services-
1. Foundation of Effective Road Image Capture through Superior Data:
Deep Learning’s pivotal role in Machine Vision necessitates the use of superior quality data. This data, marked by its accuracy and enhanced with pertinent indicators, forms the foundation for effective road image capture. It provides Machine Learning algorithms with the tools to decipher intricate patterns, thereby augmenting the precision of road image capture.
2. Increase Efficiency in Road Image Analysis through Semantic Segmentation:
Addressing the intricate challenges present in road scenarios demands the application of advanced techniques such as Semantic Segmentation, a vital element of Computer Vision. This method partitions an image into distinct segments, each symbolizing a unique object or a portion of it. This holistic approach to data annotation markedly improves the Machine Learning model’s competency in precisely analyzing and interpreting road images, thereby escalating the overall efficiency of road image capture.
3. Leveraging Top-Grade Data for Enhanced Road Image Gathering:
Acknowledging the impact of Deep Learning on Machine Vision, it becomes crucial to harness top-grade data. This data, defined by its accuracy and fortified with suitable indicators, facilitates successful road image gathering. It enables Machine Learning algorithms to decode complex patterns, thereby enhancing the accuracy of road image gathering.
Role of Machine Learning in Road Imaging Data Annotation
- Machine Learning plays a crucial role in annotating data for road imaging.
- Necessity for high-quality, accurately marked data underscores Machine Learning’s significance.
- Enriched data with relevant indicators forms the foundation for efficient road image acquisition.
- Equips Machine Learning algorithms to unravel complex patterns, enhancing the exactness of image acquisition.
- Utilization of Sophisticated Techniques like Semantic Segmentation:
- Confronting complex challenges in road situations demands the use of advanced methods.
- Semantic Segmentation, a vital component of Computer Vision, is deployed.
- Technique disassembles an image into separate segments, each representing distinct objects.
- Comprehensive approach significantly enhances the proficiency of Machine Learning models in analyzing and interpreting road images.
Challenges in Road Imaging Data Annotation
Deep Learning’s pivotal function in Road Imaging Data Annotation is highlighted by the demand for meticulously annotated, high-grade data. This enriched data, laden with pertinent markers, forms the foundation for effective road image capture. It provides Deep Learning algorithms with the tools to decipher intricate patterns, thereby enhancing the precision of road image capture.
Addressing the intricate challenges embedded in road scenarios necessitates the utilization of advanced methods such as Semantic Segmentation, a vital element of Computer Vision.
This method disassembles an image into individual segments, each embodying a unique object or a fragment of it. This exhaustive approach to data annotation considerably boosts the Deep Learning model’s competence in precisely scrutinizing and comprehending road images, thereby augmenting the overall efficiency of road image capture.
Deep Learning’s significant contribution to Road Imaging Data Annotation is emphasized by the need for precisely annotated, superior quality data. This data, infused with relevant signals, establishes the basis for efficient road image collection. It arms Deep Learning algorithms with the resources to decode complex patterns, thereby improving the accuracy of road image collection.
Future Trends in Data Annotation for Road Imaging
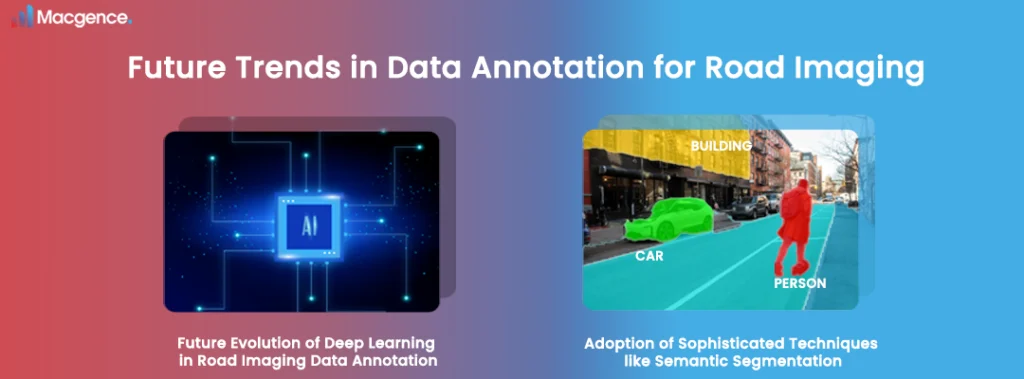
Let’s explore some future trends in data annotation for road imaging-
Future Evolution of Deep Learning in Road Imaging Data Annotation:
- Driven by the demand for exceptional quality data annotated with precision.
- Enriched data with relevant indicators lays the foundation for effective road image acquisition.
- Equips Deep Learning algorithms to interpret complex patterns, enhancing image acquisition accuracy.
Adoption of Sophisticated Techniques like Semantic Segmentation:
- Semantic Segmentation, a vital component of Computer Vision, addresses complex challenges in road scenarios.
- Breaks down images into distinct segments representing unique objects or parts.
- Comprehensive approach significantly enhances the Deep Learning model’s ability to analyze and understand road images, improving overall effectiveness of image acquisition.
Macgence Benefits & Advantages in Road Imaging Services
Macgence’s application of Deep Learning in data annotation is a testament to its commitment to innovation. This feature, when applied to road imaging, provides an advantage of high-precision data labeling. The benefit is a more accurate representation of road scenarios, which is crucial for the development of reliable AI systems in autonomous driving. By leveraging Deep Learning, Macgence has elevated the standards of data annotation in road imaging. This feature offers the advantage of automated, high-quality data labeling. The resulting benefit is a significant improvement in the local relevance of AI models, thereby enhancing their performance in real-world applications.
Conclusion:
Delving into the latest research in model training for image segmentation applications, it is clear that data labeling Machine Learning plays a pivotal role. The industry-standard techniques for optimizing data labeling and annotation in road imaging have evolved significantly, leading to more accurate and efficient Machine Learning models.
When considering data annotation Machine Learning services for road imaging, it is essential to look for providers that offer a combination of automated and manual annotation, ensuring the highest level of accuracy. Macgence, for instance, offers unique benefits and advantages in road imaging services, including automated data labeling with Machine Learning. The impact of such a data labeling service on road imaging is profound, leading to safer, more reliable autonomous vehicles.
FAQs
Ans: – Data annotation is essential because it produces high-quality, precisely labeled data that serves as the basis for efficient road picture collecting and allows Machine Learning algorithms to precisely decode intricate patterns.
Ans: – By dividing pictures into separate segments, semantic segmentation—a crucial aspect of computer vision—helps to better equip machine learning models to analyze and understand road images. This helps to overcome the complex obstacles that arise in road scenarios.
Ans: – Machine Learning algorithms can decipher complex patterns thanks to the rigorous annotation and high-quality data that Deep Learning requires.