The accuracy and usefulness of medical image annotation stand out as essential elements in the complex field of medical technology, converting static images into dynamic instruments for improving healthcare. Through the painstaking addition of information to medical imaging, this complex technique makes it possible for medical practitioners and technology to connect with vital data more effectively. This technology has far-reaching consequences, ranging from aiding in complex procedures to identifying ailments, making it a vital component of today’s medical environment.
In this article, we’ll examine medical image annotation and its several forms, including polygons, bounding boxes, and keypoints, which aid in defining and identifying particular characteristics in medical images. To illustrate the special difficulties and demands of the medical industry, such as strict data privacy regulations and the necessity for domain-specific knowledge, we will compare these specialized methods with general data annotation. In addition, we’ll look at the primary obstacles to annotation—including confidentiality and high accuracy standards—and talk about how resolving these problems improves healthcare outcomes with applications like robotic surgery and AI-assisted diagnostics.Â
What is the Medical Image Annotation?
To make medical images machine-readable, metadata must be included. This is known as medical image annotation and labeling. Nevertheless, the two differ in a few specific ways.
Annotation is the addition of extra features to a picture, including labels, bounding boxes, or segmentation masks. Machine learning models that assess and diagnose medical issues may be trained with the use of this data.
Giving an image a single label is the process of labeling it. This label might be anything as basic as normal or abnormal, or it could be something more intricate like the position and size of a tumor.
What Distinguishes Medical Image Annotation From General Data Annotation?
Identifying and annotating photos for the healthcare industry is a completely different task compared to ordinary image annotation. The following are some of the differences:
Data Accessibility
Regular photographs are normally accessible to the public or covered by conventional NDAs; medical imaging, on the other hand, is typically subject to stringent data processing agreements. The patient’s privacy is the primary concern. Medical imaging data is usually difficult to get compared to other data types.
Technical Disparities
Regular images are tiny, have only one layer, and have little amount of depth. Medical images are frequently huge, have a greater bit of depth, and contain numerous layers (slices).
Moreover, both will have distinct labeler profiles. While generalist data annotators may deal with nearly any image, medical imaging annotation necessitates the expertise of certain healthcare professionals.
These professionals are used to certain paradigms in UI and UX. Therefore, it’s important to consider if medical professionals can readily utilize the keyboard controls and user interface (UI) of a data labeling platform before selecting one.
Types of Medical Image Annotation
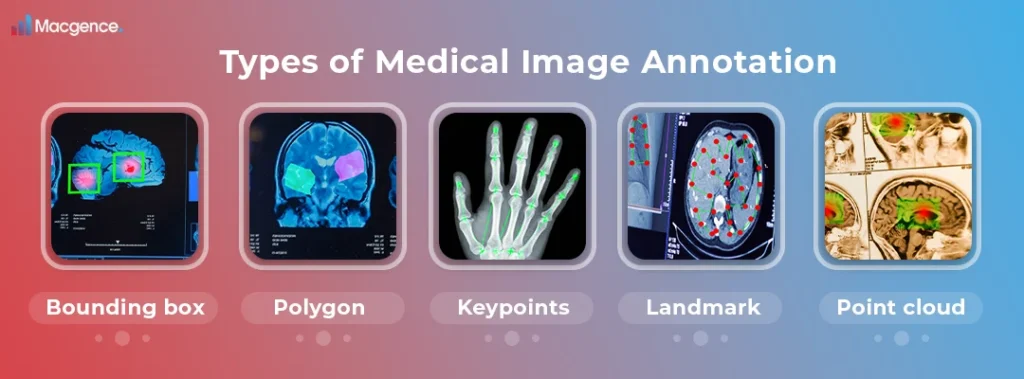
Bounding box: A bounding box in an image is a rectangular area that surrounds an item. It is the most basic and prevalent kind of annotation.
Polygon: A polygon is a closed figure used to annotate objects with irregular forms. It is composed of several line segments.
Keypoints: In an image, keypoints indicate the positions of particular features. They are frequently used to annotate tiny or challenging-to-identify items with a bounding box or polygon.
Landmark: A landmark in a photograph is a particular point of interest, such as the tip of the nose or the core of the tumor. For activities involving the alignment of two or more photographs of the same object, known as registration, landmarks are mainly utilized.
Point cloud: The 3D coordinates of an item are represented by a group of points called a point cloud. Tasks involving volumetric segmentation and registration are handled by it.
The Greatest Obstacles to Medical Data Annotation
Confidentiality
Data collected in the medical field is extremely private and governed by privacy laws. It is essential to make sure that the data is treated with rigorous privacy and security rules while utilizing a cloud platform for data labeling or outsourcing the labeling process.
Proficiency
The requirement for domain expertise for data labeling in the medical field presents another difficulty. Due to its complexity, medical data might be difficult for an inexperienced labeler to accurately annotate. This is where radiologists’ and radiographers’ training and experience are useful.
Formats
The formats used for medical imaging data differ from those used for conventional image formats. These formats are more capable and fit the requirements of medical practitioners and systems.
Medical Image Annotation Applications in Healthcare
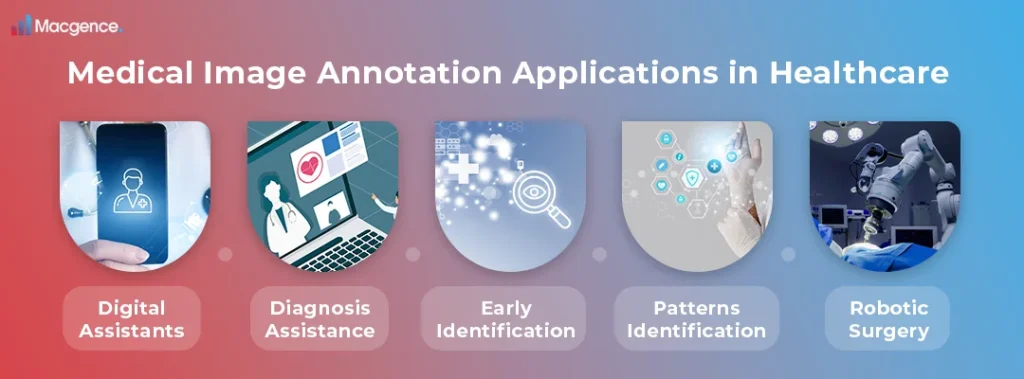
Annotating medical images may be used for more than just diagnosing and identifying illnesses. AI and ML models that have been trained on well-trained data have improved healthcare services. Here are some other uses for annotation in medical images:
Digital Assistants
AI virtual assistants are becoming more capable of delivering precise and timely information thanks to medical image annotation. It employs pre-trained data and medical image analysis to determine relevance and provide replies.
Diagnosis Assistance
AI models can help doctors diagnose patients more accurately by correcting human mistakes. It can lower execution costs while accelerating the detection of situations.
Early Identification
Identification of early biomarkers or life-threatening symptoms is crucial for early diagnosis, especially with illnesses like cancer, where a delayed diagnosis might have deadly consequences.
Patterns Identification
particularly the process of developing new drugs, pattern recognition comes particularly handy for identifying certain biological reactions to various substances through the annotation of medical images.
Robotic Surgery
AI and medical image annotation collaborate to understand intricate human body architecture and parts in robotics surgery. AI models can precisely execute surgery with the use of this data.
How Macgence Excels in Providing Medical Image Annotation Services
Macgence combines in-depth knowledge, strict security, and cutting-edge technology to succeed in medical image annotation. Medicine specialists make up their team, and they guarantee excellent annotation accuracy and clinical relevance. Since medical data is sensitive, Macgence protects patient information using secured systems and strong privacy rules. Additionally, they provide medical practitioners with specialized, easily navigable interfaces that optimize workflow efficiency without compromising functionality. Macgence is a reliable partner in enhancing patient outcomes and diagnostic procedures since it integrates AI and machine learning to assist a range of medical imaging modalities.
Conclusion:
It is impossible to overestimate the role that medical image annotation plays in advancing healthcare through technology. From robotic surgery to early illness diagnosis, this technology serves essential applications in healthcare by providing accurate and fast interpretation of medical images. It requires specialized expertise, the integration of cutting-edge technology, and adherence to stringent privacy standards—all of which Macgence skillfully satisfies. Macgence is a significant participant in the medical industry, providing reliable solutions tailored to the needs of the industry. This allows medical professionals to take advantage of digital breakthroughs to improve patient outcomes. It is therefore essential for the advancement of medical diagnosis and treatment plans to adopt such sophisticated annotation services.
FAQs
Ans: – Bounding boxes, polygons, keypoints, landmarks, and point clouds are common forms that have distinct functions for recognizing and defining elements in medical images.
Ans: – The security of extremely sensitive data must be maintained, and accuracy and domain-specific knowledge are essential.
Ans: – It improves the precision of diagnoses, helps identify diseases early, aids robotic surgery, and makes it easier to create AI-powered instruments for better healthcare delivery.