In the context of the quickly shifting landscape of AI, sensor fusion for AI models is definitely among the most promising technologies. Providing new levels of accuracy and performance. When creating datasets for AI models, a fusion of different sensor data is aimed at developing libraries of datasets. That would allow models to make more fine-grained decisions. With uses ranging from self-driving cars to intelligent medical devices, those intending to take the best advantage of AI cannot avoid sensor fusion for AI models. The following article focuses on how sensor fusion is accomplished in AI models. Its fundamental technologies, and its application and implementation in AI models, including future predictions. Buckle up, tech enthusiasts and data scientists— it’s time to consider AI’s new possibilities!
To put it simply, Sensor Fusion for AI models combines multiple sensors to create an AI model that provides more reliable and accurate information. This technique is widely used in AI model development to improve data quality and model performance. In automotive, robotics, medical and healthcare industries, sensor fusion for AI models plays a crucial role.
In the case of self-driving vehicles, we see the combination of data from cameras, LIDAR, and radar offering an enhanced awareness of surroundings. Which in turn allows a smooth self-driving process. The case is similar regarding AI-powered medical systems overly relying on AI model sensor fusion of AIs to keep a close eye on patients’ signs to enhance patient care.
The relevance of sensor fusion for AI models can’t be stressed enough. Sensor fusion allows for a different working method and has great potential as the architecture makes it possible to harness previously divergent data. Whether optimizing image recognition, improving natural language processing or predictive analytics on sensor fusion for AI models. It is the glue that holds it all together.
The Technical Side of Sensor Fusion for AI Models
The technical side of sensor fusion for AI models deals with classifying sensors predominantly used. Each type includes visual sensors such as cameras to motion sensors like accelerometers and gyroscopes. Which all add up to the understanding of the ecosystem surrounding the sensor. For instance, in the case of autonomous robots, vision sensors take pictures of the surrounding landscape. While motion sensors determine the movement and orientation during which the images are taken.
In order to obtain higher accuracy and better performance, combining output from these multiple sensors is essential. The output of all sensors must first be combined to form a single piece of information that the AI model can then comprehend. As part of this integration, algorithms are needed which can take in multiple input types and output them as one single type of data. It’s a complex task that significantly enhances data reliability and model precision.
When integrating sensor data, there are a lot of issues which have to be dealt with. Almost all developers have to deal with many problems, including variations in sensor output data, synchronization problems, and sensor data integration issues. Some solutions include advanced algorithms that effectively eliminate and synchronize issues resulting from the noise generated when integrating sensor data.
AI Models Sensor Fusion Algorithms
Sensory data integration in AI models entails the use of complex algorithms that are developed for specific purposes. One of the most recognized algorithms uses the Kalman filter, a typical approach employed when real-time processing is required, such as in navigation systems. It is also known that the Kalman filter is good at mass merging data that has been corrupted and incorporates strong guessing, which has earned its significance in the task of sensor fusion.
Another vital algorithm lies in particle filtering; it comes in handy in case there is a lot of data uncertainty in complex environments. Unlike standard approaches, particle filtering can accommodate nonlinear and non-Gaussian data, which allows for good performance in many situations.
These facilitate improvement in the performance of AI models. For example, in the case of augmented reality applications, other sensor fusion algorithms can provide a tight coupling between the virtual object and the physical world, yielding a believable experience to the end user. In agriculture, farm sensor fusion for AI models helps to do predictive analytics which recommend optimal planting strategies based on several factors such as weather and soil and plant health conditions.
Real-World Applications

This one technology has already been able to adapt in many industries, with the technology to innovate new ways of working. We can observe that autonomous vehicles use sensor fusion for AI models to better comprehend road conditions, obstacles, and traffic flow. Sensor fusion for AI models enhances vehicles’ overall safety and performance for companies like Tesla and Waymo.
In robotics, sensor fusion makes it possible for machines to perform their functions self-sufficiently and accurately engage with their environment. The robots gather information from various sensors to know the spatial arrangement of objects within the environment and make wise decisions. That is especially useful in production systems where robotic systems contribute to increased output by undertaking complicated jobs efficiently.
Another area driving sensor fusion for AI is the healthcare sector. Real-time tracking allows the fusion of wearables and medical devices. Thus providing more significant opportunities for prevention and customized treatment methods based on early providers’ intervention. Such methods bring better integration of data, which in return benefits in reaching expected goals for better diagnosis and treatment of patients.
The future of sensor fusion for AI models
In the long term, we expect technological improvements and widespread use across industries to drive the growth of sensor fusion trends for AI models. As the number of IoT devices increases, the availability of data for sensor fusion in AI models will rise. Therefore, innovators will make possible more new concepts and usages.
For AI builders and data scientists, the trends have promise and performance. More businesses are seeking sensor fusion solutions; therefore, there is excellent potential for a new body of work. However, sophisticated tools and capabilities will be essential for coping with the growing intricacy and volume of data.
The maturation of sensor fusion technology will usher in newer. Improved models that can guarantee higher accuracy and more application areas. Such will provide opportunities for city transformation, better conservation of the environment and improvements in personalized medicine.
Best Practices for Implementing Sensor Fusion for AI Models
Achieving the desired results when implementing sensor fusion calls for the following strategies. One of them ensures the data is accurate by deploying precise and trustworthy sensors. The accuracy of the AI models will depend on the sensors chosen for the specific application.
Also, it is worth considering models that can achieve better efficiency. The developers need to design the AI algorithms to process the data quickly while still retaining its accuracy. In this case, we must implement the proper processing frameworks and cloud computing services.
Another important aspect of sensor fusion is real-time data integration. As autonomous vehicles and robotics applications require the capability of data processing in real-time. Such applications also require the deployment of systems that accommodate data streaming updates.
Pulling it all Together
AI model sensor fusion is bound to change the developmental phase of AI and bring about new ideas and advancements which were never witnessed before. It assists AI models in being more resilient, accurate. Able to perform adequately in complex activities by employing multiple sensors simultaneously.
AI developers, data scientists, and technology enthusiasts should be aware of sensor fusion’s possibilities for autonomous models. A better understanding of sensor fusion is essential, irrespective of the field, whether it is autonomous cars, robotics or healthcare solutions. As it will help tap new opportunities and gain competitive advantage.
Are you ready to use sensor fusion in your projects? Don’t forget to include it in your AI models to see the changing nature of performance and accuracy. Sensor fusion, when apprehended appropriately, can trigger your innovations to reach new heights.
Frequently asked questions
Ans: – It is essential because it improves data quality by enhancing and providing a more comprehensive info range for model applications. Examples such as autonomous systems of vehicles, robotics technologies as well as the field of healthcare diagnostics. Sensor fusion is joining multiple sensor data to create augmented dense info for the AI models.
Ans: – Some challenges are variations in sensor outputs, time delays in information obtained, and the information collected outliers. Advanced algorithms designed to ensure artifacts-free data blend in the most robust signal model are crucial for developers’ implementation.
Ans: – Autonomous vehicles utilize a network of cameras for visuals. LIDAR technology and radar detect colliding positions and direct the photo. Where each degree of angular location attaches to a specific picture, creating an augmented environment model that drones use for navigation intel.
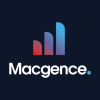
Macgence is a leading AI training data company at the forefront of providing exceptional human-in-the-loop solutions to make AI better. We specialize in offering fully managed AI/ML data solutions, catering to the evolving needs of businesses across industries. With a strong commitment to responsibility and sincerity, we have established ourselves as a trusted partner for organizations seeking advanced automation solutions.