Understanding the latest Deep Learning and Machine Learning research offers a competitive edge—enabling swift adaptation to technological advancements. It facilitates the creation of robust, efficient models, enhancing predictive accuracy in business, education, and social applications. Moreover, recent engineering case studies provide invaluable insights into practical applications, driving innovation and fostering growth.
In this article, we will explore current work on model training for panoptic segmentation systems, particularly emphasizing improvements in damaged vehicle polygon annotation. Gaining a competitive edge via familiarity with the most recent developments in Deep Learning and Machine Learning research allows for quick response to technological changes. We will examine how damage vehicle annotation methods are being revolutionized regarding accuracy and efficiency. These innovations include using Deep Learning algorithms, integrating semi-supervised Machine Learning models, active learning tactics, and sophisticated algorithms. Now, let’s explore the nuances of these developments and how they affect machine vision applications.
Ideal Techniques for Enhancing Damage Vehicle Polygon Annotation
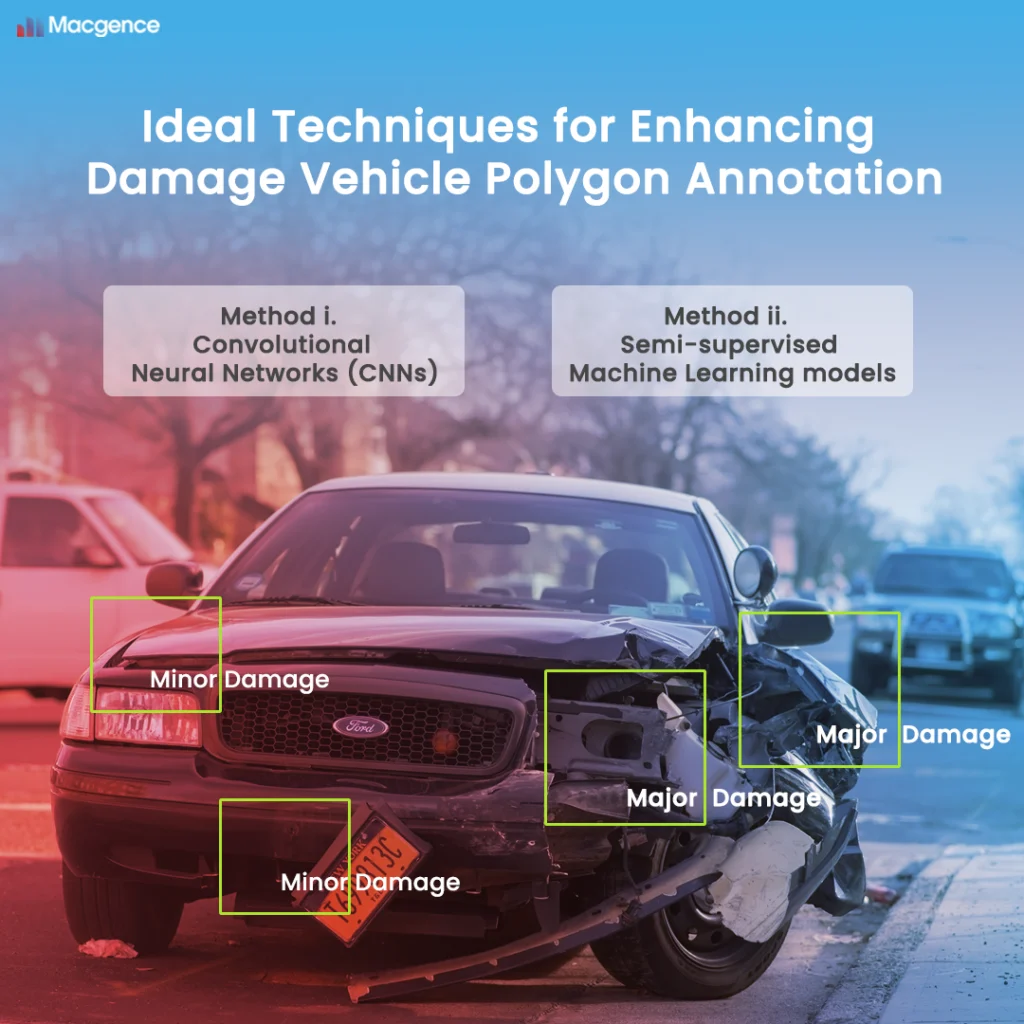
Applying deep learning methods to damage vehicle polygon annotation can significantly improve the accuracy and productivity of the annotation process. Convolutional Neural Networks (CNNs) can help distinguish and identify damaged regions in car photos. CNNs can interpret pictures in layers, which allows them to recognize complex patterns and variations in damage. This allows for a more sophisticated annotation process.
Semi-supervised Machine Learning models are another method that may be used to enhance Damage Vehicle Polygon Annotation. These models can be instrumental in scenarios with a significant diversity of damage kinds and severity levels since they incorporate labeled and unlabeled data.
Advanced algorithms for damaged vehicle polygon annotation

Advanced algorithms leverage CNNs to optimize the annotation process. CNNs effectively identify and outline damaged areas in vehicle images. The layered processing capability of CNNs enables the detection of complex damage patterns and variations.
- Enhancements through Semi Supervised Machine Learning:
- Semisupervised models utilize both labeled and unlabeled data.
- It is particularly beneficial for scenarios with diverse damage types and severity levels.
- It helps generate more thorough and precise polygonal annotations, enhancing Machine Vision applications.
- Integration of Active Learning Strategies:
- Active learning involves the ML model in the annotation process.
- ML model selects informative samples for annotation, enhancing efficiency.
- Ensures continuous learning and improvement of damage detection and classification capabilities.
- Benefits of Deep Learning Algorithms:
- Deep Learning algorithms significantly amplify the precision and speed of annotation.
- CNNs assist in pinpointing and outlining damaged areas in vehicle images.
- Recognition of intricate patterns and variations in damage enables a nuanced annotation process.
- Advantages of Semi Supervised Machine Learning Models:
- Effective in situations with a high diversity of damage types and severity levels.
- Amalgamates labeled and unlabeled data for comprehensive annotations.
- Enriches learning capabilities of Machine Vision applications.
Integration of deep learning in vehicle damage annotation
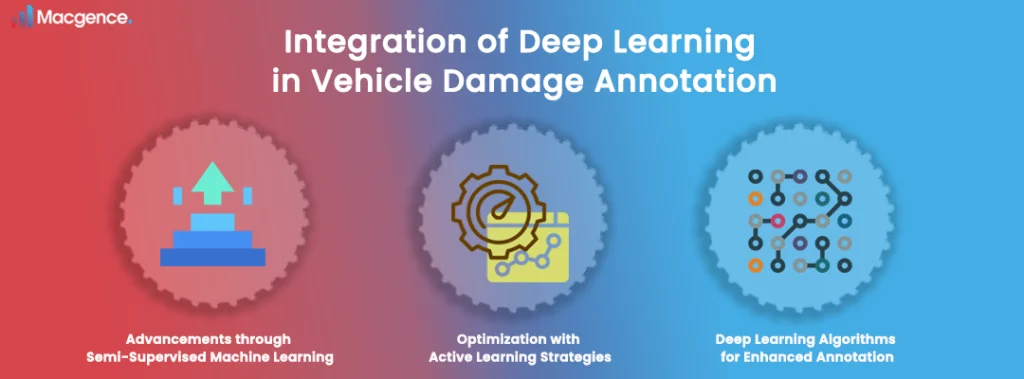
Deep Learning’s integration into Damage Vehicle Polygon Annotation transforms the process of identifying and delineating vehicular damage. The application of Convolutional Neural Networks (CNNs) enables robust detection of intricate damage patterns. CNNs’ layered image processing capabilities facilitate a detailed and nuanced annotation process, enhancing efficiency and precision in damage detection.
1. Advancements through Semi-Supervised Machine Learning:
- Further advancements in Damage Vehicle Polygon Annotation can be achieved by deploying semi supervised Machine Learning models.
- These models utilize labeled and unlabeled data, particularly beneficial in scenarios with diverse damage types and severity levels.
- Semi Supervised learning generates comprehensive and precise polygonal annotations, enhancing the learning capabilities of Machine Vision applications.
2. Optimization with Active Learning Strategies:
- Active learning strategies can optimize the Damage Vehicle Polygon Annotation process.
- By involving the Machine Learning model in the annotation process, informative samples can be selectively chosen.
- This strategy enhances efficiency and fosters continuous learning and improvement of the model’s damage detection and classification capabilities.
3. Deep Learning Algorithms for Enhanced Annotation:
- Deep Learning algorithms significantly enhance the accuracy and speed of the annotation process in Damage Vehicle Polygon Annotation.
- CNNs aid in accurately identifying and outlining damaged areas in vehicle images.
- CNNs’ layered image processing enables them to discern complex patterns and variations in damage, facilitating a detailed and nuanced annotation process.
Role of computer vision in enhancing polygon annotation

In particular, computer vision is essential for improving polygon annotation in damage vehicle polygon annotation. The ability of CNNs to interpret images in layers allows for a more intricate and subtle annotation process, which increases damage identification accuracy and speed.
Advancements through SemiSupervised Machine Learning:
- Integrating semi-supervised Machine Learning models into Damage Vehicle Polygon Annotation leads to significant advancements.
- These models utilize labeled and unlabeled data, which is beneficial in scenarios with diverse damage types and severity levels.
- Semi-supervised learning contributes to creating more accurate and comprehensive polygonal annotations, enhancing the learning potential of Machine Vision applications.
Optimization with Active Learning Strategies:
- Active learning strategies further optimize the Damage Vehicle Polygon Annotation process.
- By involving the Machine Learning model in the annotation process, informative samples can be selectively identified.
- This strategy enhances efficiency and promotes continuous learning and improvement of the model’s damage detection and classification capabilities.
Impact of high-quality datasets on damage vehicle annotation

1. Importance of High-Quality Datasets:
– CNNs’ multi-layered image processing capabilities facilitate a comprehensive annotation process, improving accuracy and speed in damage identification.
2. Advancements through Semi-Supervised Machine Learning:
– Integrating semi-supervised Machine Learning models into Damage Vehicle Annotation leads to significant advancements.
– These models utilize labeled and unlabeled data, beneficial in scenarios with diverse damage types and severity levels.
– Semi-supervised learning aids in creating more accurate and comprehensive polygonal annotations, enhancing the learning potential of Machine Vision applications.
3. Optimization with Active Learning Strategies:
– Employing active learning strategies further optimizes the Damage Vehicle Polygon Annotation process.
– By integrating the Machine Learning model into the annotation process, informative samples can be selectively identified.
– This strategy boosts efficiency and promotes continuous learning and improvement of the model’s damage detection and classification capabilities.
4. Enhanced Accuracy with Advanced Deep Learning Techniques:
– High-quality datasets combined with advanced Deep Learning techniques significantly enhance the accuracy of Damage Vehicle Annotation.
– CNNs enable a sophisticated approach to damage detection, with layered image processing facilitating a detailed and nuanced annotation process.
– This improves precision and efficiency in damage identification.
5. Advancements with Semi-Supervised Machine Learning:
– Incorporating semi-supervised Machine Learning models into the Damage Vehicle Annotation process leads to significant advancements.
– These models, utilizing labeled and unlabeled data, are beneficial in scenarios with diverse damage types and severity levels.
– Semi-supervised learning aids in creating more accurate and comprehensive polygonal annotations, enhancing the learning potential of Machine Vision applications.
Future trends in damage vehicle polygon annotation

Integrating advanced technologies like Convolutional Neural Networks (CNNs) is pivotal in the evolving realm of damage vehicle polygon annotation. These techniques enhance annotation processes, providing multi-layered image processing capabilities that boost speed and accuracy in damage detection. Emerging trends include adopting semi-supervised Machine Learning models leveraging labeled and unlabeled data for precise annotations. Active learning strategies further optimize the process by selectively identifying informative samples. Additionally, combining high-quality datasets and advanced Deep Learning techniques, particularly CNNs, promises to revolutionize annotation accuracy, enabling detailed and efficient damage identification.
Get Started with Macgence
At Macgence, we provide customized solutions to assist companies in making the most of the most recent developments in machine learning and deep learning. Our specialized advice, tailored solutions, and valuable insights help companies remain ahead of today’s quickly changing technology world.
Professional Advice: Our staff offers thorough advice on incorporating the newest Deep Learning and Machine Learning methods into your operational procedures.
Tailored Solutions: We ensure that the latest technologies are used to their fullest potential by customizing our solutions to your unique requirements.
Practical Insights: Using case studies, we provide priceless insights into practical applications that promote development and innovation.
Ongoing Support: We give you regular updates on the newest advances so you can quickly adjust to technological improvements.
Conclusion:

Model training for panoptic segmentation systems has revolutionized thanks to recent deep learning and machine learning developments. Businesses may improve the precision and effectiveness of annotation processes by using cutting-edge algorithms and active learning techniques.
Businesses may unleash new potential for development and innovation by navigating the difficulties of machine learning and deep learning with the help of Macgence services—our customized solutions and ongoing assistance guarantee that companies remain ahead in today’s cutthroat market.
FAQ’s
Ans: – Panoptic segmentation is essential for thorough scene interpretation in applications such as autonomous driving since it divides all objects in an image into semantic and instance categories.
Ans: – By automating feature extraction and hierarchical learning, Deep Learning—particularly CNNs—improves the efficiency and accuracy of segmentation.
Ans: – Semi-supervised algorithms use labeled and unlabeled data to boost scalability and annotation accuracy in panoptic segmentation.