Industrial sensor data is reshaping how industries function, providing a wealth of information to optimize operations, improve safety, and drive innovation. Pairing this rich data with machine learning (ML) offers a vast opportunity to analyze, predict, and revolutionize industrial processes. But what exactly is industrial sensor data, and how does ML unlock its full potential? This guide unpacks the essentials of how industrial sensor data and ML are used together to transform industries.
What Is Industrial Sensor Data and Why Pair it With Machine Learning?
Industrial sensor data refers to the raw information gathered by sensors deployed in machines, equipment, and industrial processes. This data can measure everything from temperature fluctuations, pressure levels, and vibrations to sound waves, infrared signals, and chemical compositions. Industries such as manufacturing, energy, transportation, and healthcare are already leveraging this data to improve efficiency and decision-making.
Machine Learning, a subset of artificial intelligence (AI), plays a crucial role in analyzing sensor data. With its ability to process massive data sets, detect patterns, and predict trends, ML gives businesses an unmatched tool to gain actionable insights—whether identifying potential equipment failures or streamlining production lines.
The Importance of Industrial Sensor Data in the Modern Era
Industrial sensor data acts as the backbone of Industry 4.0, enabling automation, smart manufacturing, and real-time monitoring. Here are some real-world examples of its impact:
Case Study #1: Predictive Maintenance in Manufacturing
A global automotive manufacturer installed sensors on assembly line machinery to monitor vibrations, heat, and operational output. Feeding this data into ML algorithms allowed them to detect early signs of wear and tear, significantly reducing unplanned downtime and repair costs.
Case Study #2: Energy Optimization
Power plants use sensors to measure energy consumption and predict load patterns. Machine learning analyzes this data to optimize energy usage in real time, reducing costs while meeting demand effectively.
Case Study #3: Enhancing Employee Safety
Some mining companies implement safety sensors connected to ML systems that predict hazardous conditions such as gas leaks or structural instability. This proactive approach saves lives while minimizing operational risks.
Understanding the Basics of Industrial Sensor Data
Before exploring ML applications, let’s understand the foundational elements of industrial sensor data:
Types of Sensors Used in Industries
- Temperature Sensors – Monitor heat levels in machinery
- Pressure Sensors – Measure force exerted by fluids or gases
- Vibration Sensors – Detect operational irregularities
- Proximity Sensors – Identify the presence or absence of objects
- Optical Sensors – Capture images or detect light intensity
Data Formats and Management
Sensor data can come in structured, semi-structured, or unstructured formats. It’s crucial for industries to employ data frameworks that aggregate, clean, and standardize this raw information for accurate ML insights.
Challenges and Opportunities with Sensor Data in ML
While the combination of industrial sensor data and machine learning offers enormous potential, it is not without its hurdles. Here are the challenges and accompanying opportunities:
Challenges:
- Data Quality Issues: Noise, missing values, or irregular frequencies can reduce data reliability.
- Infrastructure Costs: Setting up and maintaining IoT sensors and ML models can be costly, especially for small organizations.
- Skill Gaps: Experts skilled in both industrial operations and data science are in high demand but scarce.
Opportunities:
- Improved Data Preprocessing Tools: Companies like Macgence are providing curated, high-quality datasets to overcome data collection hurdles for AI/ML models.
- Growth of Partnerships: Cross-industry partnerships can make advanced AI solutions scalable and cost-efficient.
Best Practices for Implementing ML with Industrial Sensor Data
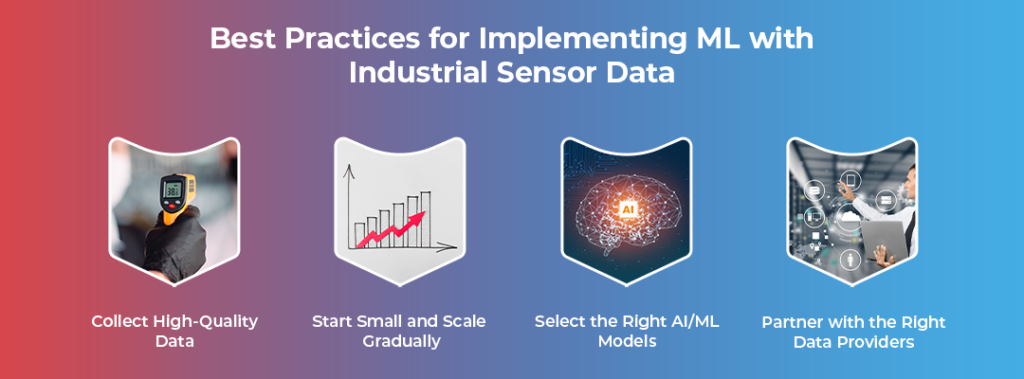
To effectively integrate ML with your industrial sensor data, follow these best practices:
1. Collect High-Quality Data
Ensure your sensors are well-calibrated, and use preprocessing techniques like filtering and anomaly detection to clean your data.
2. Start Small and Scale Gradually
Begin with a single use case (like optimizing one manufacturing line) before rolling out ML solutions across your organization.
3. Select the Right AI/ML Models
Choose models that fit your needs—whether supervised learning for predictive analytics or unsupervised learning for anomaly detection.
4. Partner with the Right Data Providers
Leverage services like Macgence, which specialize in providing curated, domain-specific datasets designed for building AI/ML models.
Future Trends in Industrial Sensor Data and ML
The future of industrial operations will likely revolve around seamless AI integrations. Here’s what’s on the horizon:
1. Edge AI
Processing sensor data directly on devices (“at the edge”) will reduce reliance on cloud infrastructure, enabling faster decision-making.
2. Digital Twins
ML-powered virtual replicas of physical systems will offer real-time monitoring and testing of industrial processes, reducing risks and costs.
3. Autonomous Operations
With sensor data and ML working together, fully autonomous factories and supply chains may become a reality, revolutionizing productivity.
4. Ethical AI in Industry
The adoption of responsible AI systems, mitigating bias and ensuring transparency, will remain a key priority.
Harness the Power of Sensor Data and AI Today
Industrial sensor data and machine learning have the potential to redefine industries by improving efficiency, enhancing safety, and optimizing processes. If your organization is ready to take the leap, start by working with a trusted data provider like Macgence—offering datasets crafted to create impactful AI/ML models.
Explore the possibilities waiting for your business with Macgence. Learn more and transform your operations today.
FAQs
Ans: – Macgence provides high-quality, curated datasets spanning multiple industries to help businesses build robust AI/ML models.
Ans: – Macgence offers data cleansing, preprocessing, and transformation services to standardize sensor data for seamless ML model integration.
Ans: – Macgence specializes in providing customized datasets tailored for industrial applications, ensuring accuracy, consistency, and scalability in AI/ML systems.
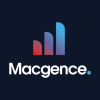
Macgence is a leading AI training data company at the forefront of providing exceptional human-in-the-loop solutions to make AI better. We specialize in offering fully managed AI/ML data solutions, catering to the evolving needs of businesses across industries. With a strong commitment to responsibility and sincerity, we have established ourselves as a trusted partner for organizations seeking advanced automation solutions.