An average human observes the things and events in the surroundings. Depending on the purpose, we can differentiate between objects, determine their distance from us and other objects, and spot errors. Similarly, computer vision training data allows AI-powered machines to train themselves to perform these tasks. The machines achieve this through cameras, algorithms, and data. However, computers, unlike humans, do not exhaust. You can train computer vision-powered machines to analyze thousands of production assets or products in minutes. Computer vision is paving its way in every sector, from manufacturing to healthcare and traffic flow analysis. This blog will dive deep into understanding computer vision, its workings, advantages, and importance.
What is computer vision training data?
Computer vision training data refers to the photos, videos, or visible media used to train computer vision models. These models discover ways to recognize patterns and features within the data, including edges, textures, shapes, and objects, to solve numerous computer vision tasks like object recognition, segmentation, tracking, registration, and more. The quality, quantity, and variety of the training data drastically affect the performance of the resulting computer vision models. Hence, carefully selecting and preparing training data is crucial in developing computer vision models.
How does computer vision work?
Computer vision services and solutions use input from sensing devices, artificial intelligence, machine learning, and deep learning to replicate how the computer vision system works. Computer vision training data runs on algorithms trained on massive amounts of visual data or images in the cloud. They recognize patterns in this visual data and use those patterns to determine the content of other images.
With the help of pre-programmed algorithmic frameworks, a machine learning system may automatically learn about visual data interpretation. The model can learn to distinguish between similar pictures if it is given a large enough dataset. Algorithms allow the system to remember independently and replace human labor in tasks like image recognition.
Computer vision training data aids machine learning and deep learning models in understanding by dividing visuals into smaller sections that may be tagged. With the help of the tags, it performs convolutions and then leverages the tertiary function to make recommendations about the scene it is observing. With each cycle, the neural network performs convolutions and evaluates the integrity of its recommendations. And that’s when it starts perceiving and identifying pictures like a human.
Advantages of Computer Vision Training Data
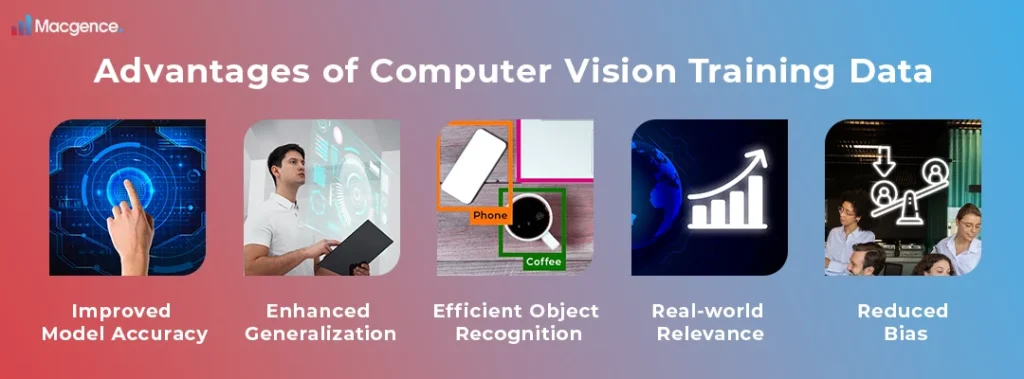
There are numerous advantages of Computer vision training data, some of which are mentioned below:
1. Improved Model Accuracy:
High-quality computer vision training data contributes to the accuracy of computer vision models. Precise and well-annotated data ensures that models learn to understand and interpret visual information more precisely.
2. Enhanced Generalization:
Diverse and representative training data allows computer vision models to generalize well to various scenarios. This allows the models to perform correctly on real-world records beyond the training set, making them more solid and versatile.
3. Efficient Object Recognition:
Well-annotated computer vision training data enables efficient object recognition. Models skilled in complete datasets can accurately identify and categorize items within photos or videos, permitting applications in diverse domains, which include autonomous vehicles, healthcare, and production.
4. Real-world Relevance:
Quality computer vision training data displays real-world scenarios, ensuring computer vision models uncover the complexities and variations present in practical applications. This real-world relevance is vital for models to perform correctly in numerous environments.
5. Reduced Bias:
Carefully curated and diverse training data helps mitigate biases in computer vision models. Biases can rise if the training data is not representative, leading to skewed outcomes. High-quality data targets minimize these biases and promote fair and unbiased model predictions.
Why is Computer Vision so necessary?
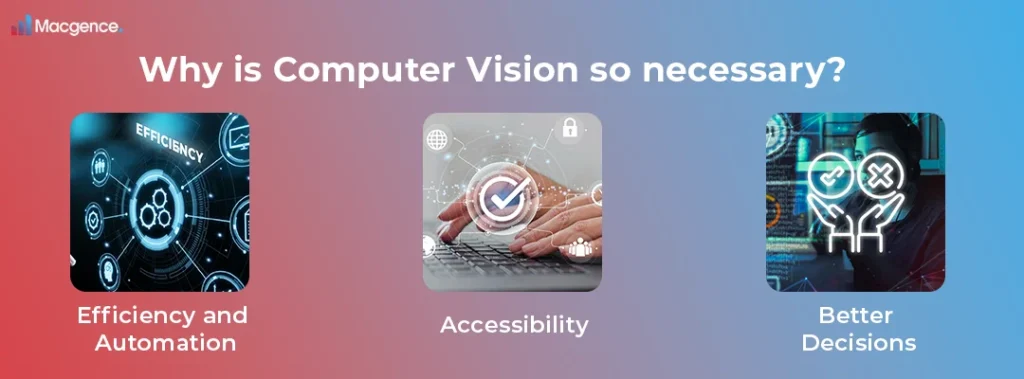
The main reasons why computer vision is deemed necessary are:
Efficiency and Automation
Computer vision enables automated visual tasks, minimizing human intervention. Hence, various processes, including data analysis, logistics, and manufacturing, have become significantly more efficient, streamlined, precise, and capable.
Accessibility
Advanced computer vision training data seamlessly facilitate the creation of broader digital environments. They assist visually impaired individuals by enabling text-to-speech capabilities and providing detailed descriptions of images.
Better Decisions
Computer vision can give companies data analytics and real-time insights to support their decision-making. For example, Businesses can use computer vision to personalize their offerings by considering the tastes and actions of their customers.
How can Macgence enhance your Computer Vision Models?
Macgence offers customized tailored solutions for your specific needs; here is how Macgence can give you an advantage over others:
Unparalleled Diversity in Training Data
Macgence takes pride in curating diverse and extensive Computer vision training data. By encompassing a wide range of scenarios, objects, and environments, Macgence ensures that your models are exposed to real-world complexities. This diversity enhances the robustness of your models, making them more adept at handling various situations.
Data Annotation Excellence
Accurate annotation is crucial for the effectiveness of Computer Vision models. Macgence excels in data annotation, ensuring that each piece of data is meticulously labeled. This precision contributes to developing models with a deep understanding of the visual information they process, enhancing their overall accuracy.
Efficient Resource UtilizationMacgence’s computer vision training data solutions enable efficient resource utilization for training Computer Vision models. By providing precisely annotated data that aligns with your project requirements, Macgence minimizes resource wastage and accelerates the training process. This efficiency translates to cost-effectiveness and quicker model deployment.
Conclusion
In conclusion, the journey through Computer Vision has illuminated the transformative impact it holds on the field of artificial intelligence. From the limited understanding of context to the dependency on the quality of computer vision services and solutions along with computer vision training data, handling variability, and the need for common-sense reasoning, these hurdles underline the evolving nature of Computer Vision.
FAQs
Ans: – Computer Vision is a field of study that enables machines to interpret and understand visual information from the world, similar to human vision.
Ans: – Facial recognition technology uses computer vision to identify specific people in photos and videos. In its lightest form, companies such as Meta or Google use it to suggest that people tag in pictures, but law enforcement agencies can also use it to track suspicious individuals.
Ans: – With the help of advanced cameras and picture processing algorithms, computer vision systems for facility management can examine photographs and videos of large public spaces. This provides precious insights into the operations of the facility.