The search for increasingly complex, effective, and precise artificial intelligence (AI) models is never-ending in the quickly developing field of AI. The state-of-the-art methods of panoptic segmentation and careful data labeling annotation are essential to this endeavor. These techniques serve as the cornerstones of contemporary AI training, providing the foundation for the creation of models capable of navigating the intricacies of real-world settings and precisely interpreting intricate facts. It is crucial to remain at the forefront of deep learning (DL) and machine learning (ML) research as AI permeates every aspect of business and daily life, changing everything from social apps to educational techniques. This environment of constant invention and application provides firms and researchers with the necessary competitive advantage.
We’ll explore the most recent R&D advances in AI training in this post, with a particular emphasis on data labeling annotation and panoptic segmentation. Our goal is to investigate how these state-of-the-art technologies are being used to augment AI’s capabilities. We will cover topics such as the subtleties of 2D bounding boxes, best practices for annotation services in AI and ML, and the importance of annotations in computer vision. Our objective is to address the tactics and issues in data labeling and annotation, and to offer insights into the cutting-edge approaches influencing AI’s future. With a focus on providing professionals and enthusiasts interested in the scientific developments driving the AI revolution with a thorough understanding of the challenges and achievements of AI development, this exploration aims to provide AI practitioners with the information necessary to capitalize on these advancements.
Best Practices for Utilizing Annotation Services in AI ML Text Annotation 2D BB
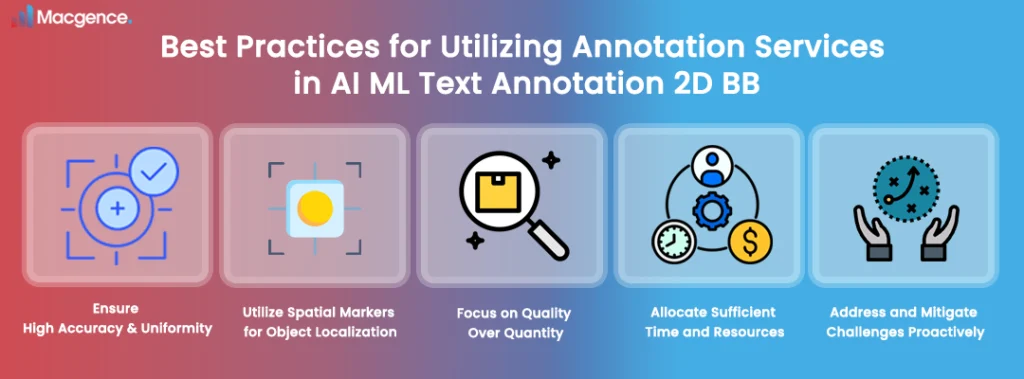
1. Ensure High Accuracy and Uniformity: Given the critical importance of data accuracy and consistency in training ML models, it’s vital that annotation services maintain an exceptional level of precision across all labeled data. This minimizes the risk of introducing biases or errors into the AI systems.
2. Utilize Spatial Markers for Object Localization: In computer vision, especially in tasks like polygon annotation and semantic segmentation, using spatial markers such as polygons can greatly aid algorithms in accurately localizing and differentiating objects within 2D images. This practice is essential for applications requiring high precision in object detection and recognition.
3. Focus on Quality Over Quantity: As AI technologies advance, the quality of data labeling and annotation is of utmost significance, despite the volume of data being significant. When training with higher amounts of poorly annotated data, machine learning models will be less robust and more dependable when each piece of data is precisely labeled.
4. Allocate Sufficient Time and Resources: Acknowledge that categorizing and annotating high-quality data takes a lot of effort and resources. To get the required degree of consistency and quality in the data needed to train AI models, enough time and resources must be set aside for this procedure.
5. Address and Mitigate Challenges Proactively: Recognize the difficulties that come with labeling and annotating data, such as the requirement for high accuracy and the possibility of discrepancies. Tackling these issues may be lessened by putting strict quality control procedures in place and giving annotators ongoing training.
By putting these best practices into practice, AI and ML projects may greatly increase the efficacy of annotation services, especially when it comes to text annotation and 2D bounding box labeling. This will boost the overall performance and dependability of the AI models that are produced.
Optimal strategies for AI ML text annotation services
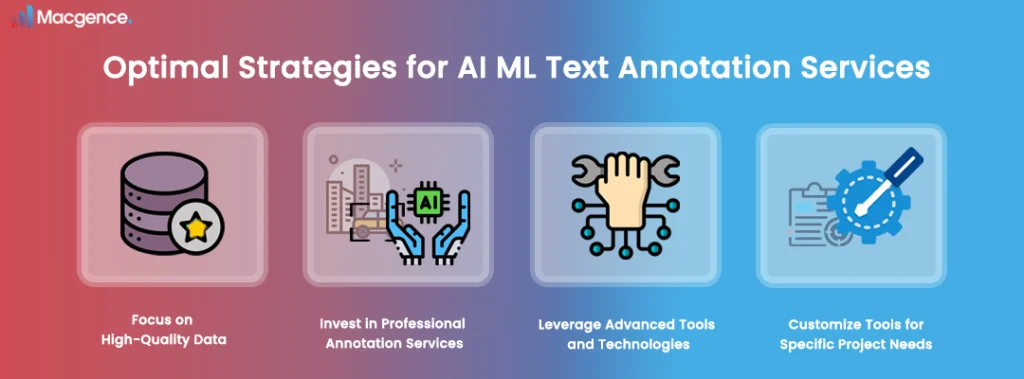
1. Focus on High-Quality Data: Make sure the data being annotated is of the best caliber possible since machine learning models that function well are built on precisely labeled data. Give accuracy top priority throughout the annotation process to facilitate the creation of trustworthy AI applications.
2. Invest in Professional Annotation Services: Utilize professional annotation services capable of providing the necessary level of detail and accuracy. This investment is crucial for organizations aiming to leverage the latest AI technologies effectively.
These tactics emphasize how crucial it is to have high-caliber, expert services and cutting-edge technology when it comes to text annotation services that are optimized for AI and machine learning.
2D bounding box: A technical perspective in AI ML text annotation
A look at the technical details of 2D bounding box in AI ML text annotation reveals that it is a method that uses rectangle shapes to contain text. This approach is very useful for computer vision problems since it helps with accurate object recognition and localization in two dimensions. The bounding box technique, which gives computers a structured framework to learn and comprehend spatial interactions, is essential to the creation of reliable machine learning models.
Practically speaking, 2D bounding box annotation is a laborious procedure that demands a high level of precision. To make sure that the algorithms can recognize and understand the data appropriately, the bounding boxes surrounding the target text must be precisely constructed dependability. When training models for intricate tasks like object identification, picture recognition, and semantic segmentation, this degree of detail is essential. Machine Learning models operate with great accuracy and dependability because of this precision.
The 2D bounding box technique offers substantial advantages in AI ML text annotation, despite its technical complexity. By giving Deep Learning models a concise, organized depiction of the data, it makes training them more effective. It also makes it possible to incorporate sophisticated annotation tools, which automate the procedure and preserve high accuracy levels. Consequently, the 2D bounding box approach is a potent weapon in the toolbox of researchers and developers of artificial intelligence, propelling the development of AI technologies.
Why choose Macgence?
Delving into the intricacies of data labeling for text annotation, Macgence emerges as a global leader in providing AI training data services. Their proficiency in handling AI training data, coupled with advanced technology and AI-driven tools, simplifies the complex task of data collection and generation. Catering to a diverse clientele, including small to medium-sized businesses, startups, corporations, and educational institutions, Macgence’s services extend beyond borders, offering localized and globalized insights from data.
Macgence’s prowess in AI and Machine Learning project preparation, development, implementation, and deployment is evident in their comprehensive product line of AI training data. Their services encompass both training and testing data, ensuring a robust data pipeline for AI initiatives. Trusted by global giants such as Amazon and Google, Macgence’s data annotation services are instrumental in enhancing the precision of Natural Language Processing and Machine Learning, thereby driving innovation and accelerating time-to-market for businesses.
Conclusion:
To sum up, the future of artificial intelligence will be greatly influenced by the ongoing developments in AI training and data labeling annotation services. The advancement of methods like panoptic segmentation and the more sophisticated approach to data annotation highlight the need for increasingly complex, precise, and effective artificial intelligence models. This evolution represents a turning point in our quest to develop AI systems that are not only intelligent but also dependable and flexible in the face of challenging real-world situations, highlighting the significance of accurately annotated high-quality data and the strategic application of expert knowledge in domain-specific annotation.
FAQs
Ans: – Semantic and instance segmentation are used in panoptic segmentation to identify and classify individual pixels in an image, which is essential for AI algorithms to comprehend complex scenes.
Ans: – Accurate and consistent data is used to train AI models by precise data annotation, which greatly improves the models’ dependability and efficiency in practical settings.
Ans: – Experts are aware of the subtleties in their field, which enhances the precision of annotated data—especially when dealing with intricate jobs that call for in-depth expertise.