How to Collect the Right Training Data for Neuro Monitoring
It is right to say that in the walk of life, including health care, competition is on the rise and so is the need for updating oneself. Both the neuroradiologist and a data scientist can relate to the applicability of this trend as training data for neuro monitoring has emerged as one of the focal points. This blog shall outline the importance of training data for neuro monitoring, some issues which were confronted and ways in which those were resolved. Furthermore, the performed activities by the data scientists will be demonstrated and some other best practices, examples, and a vision of the future will be shared.
Introduction to Training Data for Neuro Monitoring
Training data for neuro monitoring systems can be analyzed as a critical input for artificial intelligence or machine learning applications relevant to the domain. Neuro monitoring is monitoring brain activity and focusing on brain functions or neurological functions by studying them over time, often in real-time. In the context of a neuro-monitoring system, there is a strong need for the precisions of the system to evolve to assist in the diagnosis process and enhance the treatment of the patients; thus, training data is essential for developing appropriate algorithms.
However, it is essential to note that collecting and processing this data is anything but easy. This raises several issues such as upholding privacy of the data as well as bias. However, the right strategies can help counter such barriers, thus aiding in developing advanced neuro-monitoring systems.
Grasping the Fundamentals
Data representing neuro monitoring is the most crucial component of training and policy earning as it is the foundation of accuracy. However, what exactly does that mean in healthcare? According to this, the sufficient and high-quality training data for neuro monitoring has to be multi-modal, meaning that it has to include classes of patients suffering from different neuromuscular diseases and patient populations. This diversity allows the algorithms created to be developed across a broad spectrum of use cases.
The data must be well-labelled and validated to enhance accuracy. It is using unlabeled or poorly labelled data results in unreliable algorithms that could result in women receiving the wrong diagnosis. Thus, it is essential to put more emphasis on the data collection and labelling processes in detail.
Challenges and Solutions
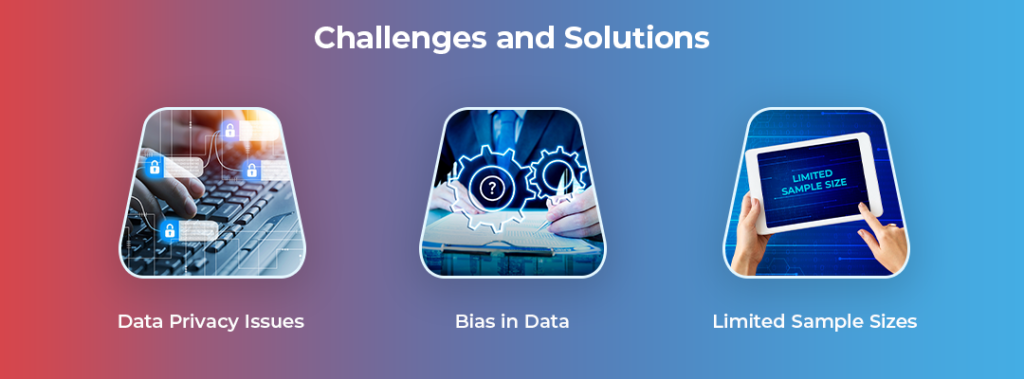
Due to data privacy issues, getting training data for neuro monitoring is an uphill task. Patient data is quite sensitive, and any violation of confidentiality can have severe consequences. Some measures to curb this issue include implementing robust data protection measures and utilizing anonymized data whenever possible.
Another major challenge is the bias present in the data. The algorithms built based on inadequate diversity of the training datasets may also have the same risk. In this regard, health professionals have to ensure that the datasets they are using adequately represent the population.
Finally, the number of sample sizes may also limit the effectiveness of neuro-monitoring algorithms. Healthcare centres can address this challenge by coming together to share their data, creating more extensive and more heterogeneous datasets.
Makeup Of Data Scientists.
Data scientists form the core of neo-monitoring since improvement in the application’s training data relies on them. They look after the development and improvement of algorithms enabling the analysis of large volumes of neuropsychological records. Their understanding of dealing with and interpreting neuro-monitoring training data extensively enhances quality and reliability over a broad range of applications.
Data scientists are also applying fundamental changes in neuro-monitoring with artificial methods like deep learning and networks. Such approaches allow the building of better models to identify even more complex non-standard processes within the neural data.
Research Implications.
To achieve this aim of enhanced quality of neuro monitoring, data scientists must also ensure that the prerequisites established in the case of collection of the neuro monitoring training data are in place. That includes, among other things, clear guidelines about data collection procedures, data labelling and validation. It is also essential to regularly examine and revise the training dataset to ensure it is up-to-date and relevant.
In addition, this would encourage the joint participation of neurologists and data scientists, resulting in richer datasets and better inferences. Both sides approach the table with their perspectives on what they need from the data and how they will use it for neuro monitoring.
Case studies
Real-life examples provide a good understanding of the significance of the training data in the context of neuromonitoring. A great example here was the prediction of the time an individual suffering from epilepsy would have a seizure using machine learning algorithms. After spending considerable time analyzing the training data specific to neuro monitoring, the system got as high as eleven nines in the amount of misclassification, all of which are excellent for patient outcomes.
Another focus area in this case was detecting Alzheimer’s disease at its early stages using AI. In this case, the training data for neuro monitoring revolved around imaging and scans as well as past records of the patients that made the algorithm train to pick up minute details that a clinician wouldn’t be able to appreciate. Detecting this at an early stage allows for an intercept that can slow the disease’s development rate.
Future outlook
There are good prospects for the future of training data, specifically in the context of neuromonitoring. New technologies, including artificial intelligence and big data analytics, promise to change how healthcare professionals manage and treat neurological diseases and disorders. It would mean more effective treatment and better patient results through such improvements.
New trends like telemedicine and wearable technology are affecting neuro monitoring and many other areas. These new inventions increase the amount of training data for the algorithms, thereby improving their quality and widening the range of neuro-monitoring applications.
Conclusion
Neuro monitoring as a field presents numerous challenges, especially in practice. However, this area has the potential to transform how practitioners treat patients. By addressing these challenges and implementing good practices, healthcare professionals can ensure that they utilize this data to improve the diagnosis and treatment of neurological illnesses.
And the future holds even more amazing technology, news, and inventions for which the elastic and aware will be the best. To all those wishing to pursue this field further, participating in ongoing research and collaborations is a significant next step.
You Might Like
February 28, 2025
Project EKA – Driving the Future of AI in India
Artificial Intelligence (AI) has long been heralded as the driving force behind global technological revolutions. But what happens when AI isn’t tailored to the needs of its diverse users? Project EKA is answering that question in India. This groundbreaking initiative aims to redefine the AI landscape, bridging the gap between India’s cultural, linguistic, and socio-economic […]
March 24, 2025
Natural Language Generation (NLG): The Future of AI-Powered Text
The ability to generate human-like text from data is not just a sci-fi dream—it’s the backbone of many tools we use today, from chatbots to automated reporting systems. This revolution in artificial intelligence has a name: Natural Language Generation (NLG). If you’re an AI enthusiast or a tech professional, understanding NLG is essential for keeping […]
March 24, 2025
HITL (Human-in-the-Loop): A Comprehensive Guide to AI’s Human Touch
The integration of Artificial Intelligence (AI) in various industries has revolutionized how businesses operate. However, AI is not infallible, and many applications still require human intervention to enhance accuracy, efficiency, and reliability. This is where the concept of Human-in-the-Loop (HITL) becomes essential. HITL is an AI training and decision-making approach where humans are actively involved […]
March 7, 2025
Data Annotation – And How Can It Build Better AI in 2025
In the world of digitalized artificial intelligence (AI) and machine learning (ML), data is the core base of innovation. However, raw data alone is not sufficient to train accurate AI models. That’s why data annotations comes forward to resolve this. It is a fundamental process that helps machines to understand and interpret real-world data. By […]