AI Accuracy with Data Validation Services
Are your AI models backed by meticulously validated data? If not, Let’s Connect.
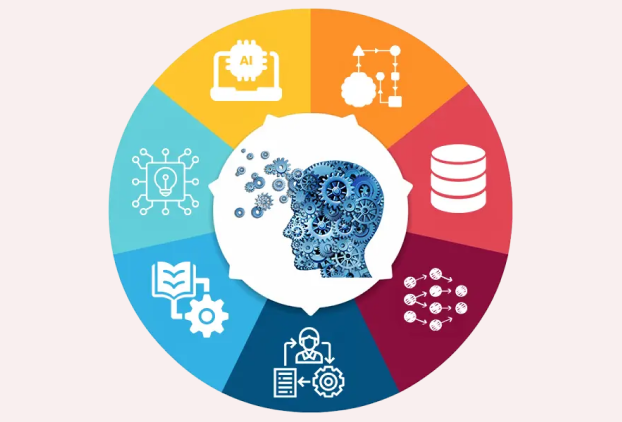
Welcome to Macgence's AI Data Validation Services
In the ever-evolving landscape of ML, the need for AI Data Validation Services arises. Consequently, Macgence stands as a beacon of innovation and excellence. Specializing in cutting-edge Data Validation Services, we meticulously craft solutions that elevate your AI models to new heights of resilience, precision, and adaptability in the face of real-world complexities. Moreover, our expert team excels in the nuanced art of model validation, data validation, and continuous monitoring. Thus, we ensure that your AI solutions seamlessly align with evolving business objectives.
Understanding Model Validation
Model validation is the cornerstone of reliable AI solutions, encompassing various crucial aspects as below:

Clean Model
Inputs
We rigorously validate information for accurate model input. Consequently, we establish a foundation for precise predictions and informed decisions.
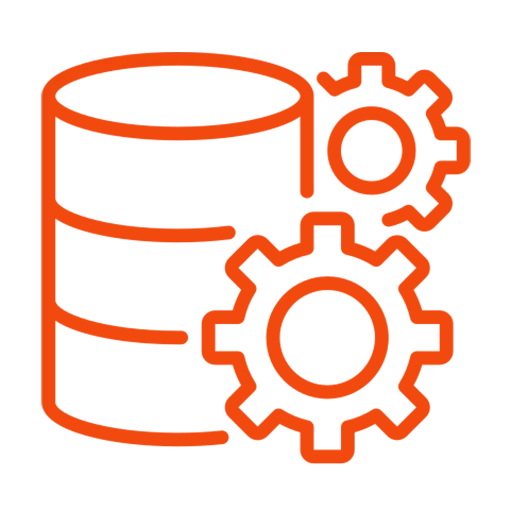
Representative Training Dataset
Using datasets that mirror real-life scenarios, we validate the model's training data. As a result, we ensure it captures diverse and relevant patterns.

Analyzing Model Performance
We rigorously test the model to check how well it works in different situations and conditions. Consequently, this process also provides valuable insights.

Stress
Testing
Our stress testing methods assess the model's resilience under pressure and extreme situations. Thus, we guarantee its stability in critical moments.

Robust Output
Analysis
We scrutinize the model's predictions to verify their accuracy and consistency. Consequently, we ensure dependable and trustworthy results for users.

Continuous
Adaptation
Ongoing evaluation and updates enable the model to adapt to evolving conditions. As a result, it maintains relevance and effectiveness over time.
Building a Strong Foundation with Data Validation
Our meticulous data validation processes ensure the data used for model training is
accurate and reliable:
Find and Eliminate
Duplicates
We identify and eliminate duplicate entries from datasets to ensure data integrity. This process prevents any inaccuracies, and maintain reliable results across diverse datasets for more accurate insights.
Authentic Data
Assurance
By validating the accuracy and relevance of data, we ensure that it accurately reflects real-world scenarios. This guarantees that the model is more effective, improving decision-making and predictive outcomes.
Addressing
Data Drift
We dynamically adjust models to account for changes in real-world data, ensuring sustained accuracy. Our approach effectively manages evolving data patterns, keeping models relevant and up to date over time.
Validation
Techniques
Our advanced techniques, including dataset splitting, duplicate sample elimination, data structure comparison, and data drift mitigation, effectively fortify the reliability, ensuring consistent model performance.
Expertise and
Diversity
With years of experience delivering expert crowdsourcing services, we efficiently tap into our global-local network of specialists. This ensures that ads and content are assessed with precision and diversity.
Confidentiality and
Security
Our platform prioritizes security and data quality, ensuring privacy while maintaining exceptional standards through validation and a seniority system. We safeguard confidentiality, providing secure data.
Approaches to Validate Machine Learning Models in Production
We employ sophisticated methods to validate AI models and ensure their ongoing success:

K-fold Cross-Validation Method
Enhancing model generalization involves testing variations; consequently, it ensures adaptability to diverse situations and improves overall performance in real-world applications.
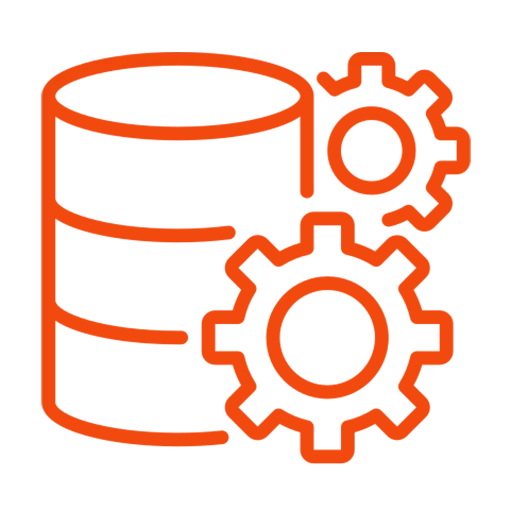
Leave-One-Out Cross-Validation Method
Evaluating individual components of the model guarantees that, consequently, each element functions effectively and contributes to the overall performance of the system.

Random Subsampling Validation Method
Utilizing random changes effectively assesses model robustness, thus ensuring its strength in unpredictable conditions and enhancing its overall reliability.

Time-Series Cross-Validation Method
Addresses temporal variations adeptly in data, thus aligning the model with evolving real-world scenarios, ensuring its continued effectiveness and accuracy over time.

Stratified K-fold Cross-Validation Method
Balances data representation effectively, thus ensuring that every component is adequately considered, even in imbalanced datasets, which promotes fair and accurate model performance.

Bootstrapped Cross-Validation Method
Enhances model robustness through effective resampling techniques, thereby ensuring reliable validation across diverse production scenarios and improving overall performance.
Continuous Model Validation and Monitoring
We emphasize the importance of continuous validation and monitoring for sustained success:

Detecting Drift
Enterprise AI is useful for creating smart chatbots that help solve queries for customers. Hence it is easier for businesses to provide quick and helpful support.
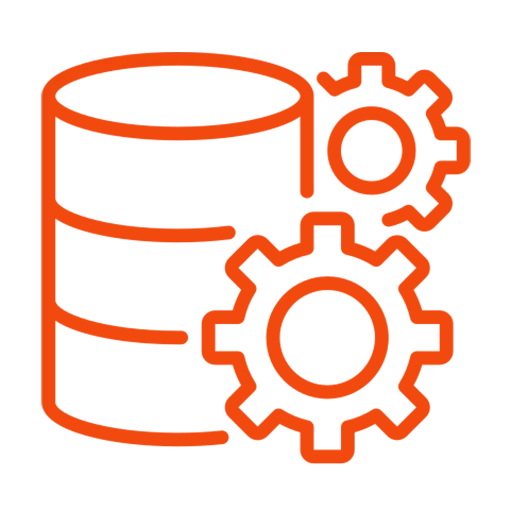
Performance Evaluation
Continuous monitoring therefore allows for a prompt response to declining accuracy, ultimately ensuring consistent performance that remains aligned with business objectives.

Early Issue Detection
Timely identification of biases, inaccuracies, or unexpected patterns therefore enables swift corrective actions, ultimately preventing negative consequences.

Ensuring Business Goals
Strategically aligning AI performance metrics with business goals ensures informed decision-making, as it provides the reliable insights and analytics for your model.

Customer Satisfaction
Customer-facing applications benefit from accurate AI/ML models, which elevate the satisfaction to unprecedented levels and enhance the customer engagement.

Dynamic Adaptation
Adaptive learning in AI boosts performance by dynamically adjusting to user preferences, thus ensuring continual improvement and personalized excellence.
Real-life examples of validation methods
Healthcare Diagnostics
In healthcare diagnostics, precision is critical. Validated models use advanced algorithms and machine learning to analyze vast medical datasets. This enables accurate disease identification, predictive insights, and optimal treatment planning, significantly improving patient outcomes through reliable, timely diagnoses.

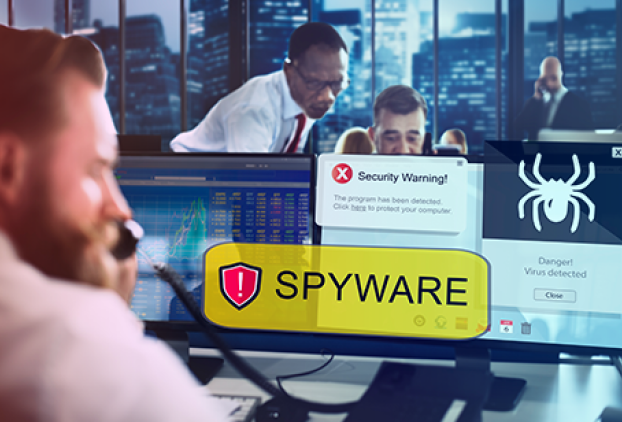
Online Banking Fraud Detection
In online banking, fraud detection models are essential. Validated models analyze transaction patterns in real-time, leveraging advanced algorithms. Through rigorous validation processes, these models promptly identify and flag anomalous behaviors, ensuring early detection of fraudulent activities and securing transactions.
Autonomous Vehicles
Autonomous vehicle decision-making relies on validated models, utilizing extensive testing, simulations, sensor data, and machine learning algorithms. These models adapt to real-time environments, excelling in collision avoidance, traffic rule adherence, and seamless navigation, ensuring safety and reliability on the road.
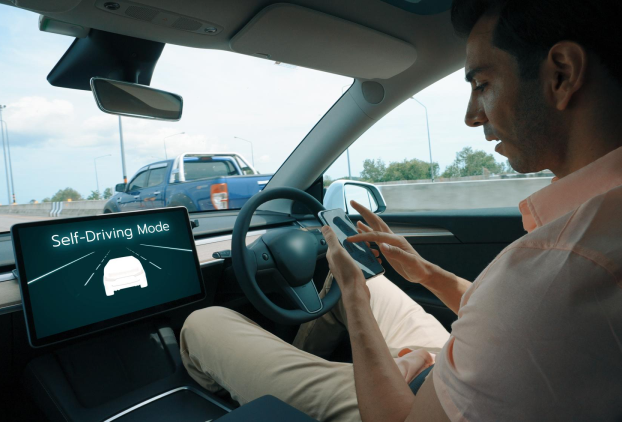
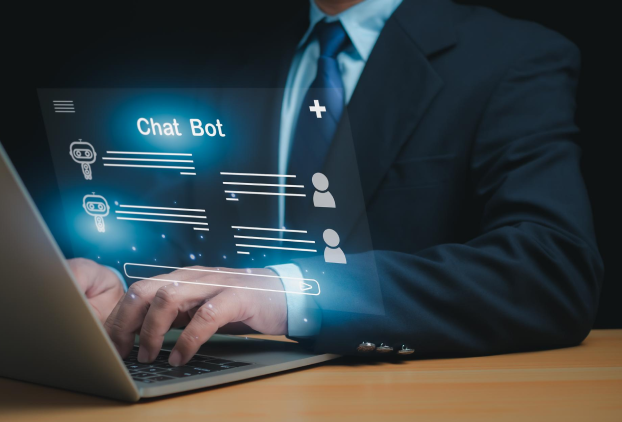
Customer Support Chatbots
Customer support chatbots leverage validated models based on natural language processing, driving efficiency. These models undergo rigorous validation, understanding and responding to queries accurately. Their learning capabilities ensure ongoing improvements, making them reliable sources for resolving customer issues effectively and quickly.
Who Can Benefit from Macgence’s Data Validation Services?
Automotive
Macgence ensures the accuracy of sensor and performance data for autonomous vehicles and ADAS systems. We validate environmental inputs and vehicle metrics, ensuring safety, optimal navigation, and transportation system efficiency.
Healthcare
In healthcare, we validate patient records, medical imaging, and diagnostic results. Our services ensure data consistency, enabling quicker, more accurate diagnoses and treatment decisions, ultimately improving patient outcomes and healthcare efficiency.
Retail
Macgence validates customer, inventory, and transaction data for retail businesses. This improves recommendation systems, personalized marketing, and inventory management, ensuring data quality and driving growth in the digital marketplace.
AR/VR
For AR/VR applications, we validate spatial data, 3D models, and object interactions. Our services enhance gesture recognition, object tracking, and environmental mapping, delivering accurate, realistic virtual environments for enhanced user experience.
Geospatial
In geospatial industries, we validate LiDAR, satellite imagery, and GIS data for applications in urban planning, land analysis, and disaster management. Our trusted data supports better decision-making, sustainable practices, and resource management.
Banking & Finance
Macgence’s data validation ensures accurate financial records, including transactions, customer data, and statements. We help improve fraud detection, risk management, and customer profiling, ensuring data security, efficiency, and regulatory compliance.
Why Choose Macgence for Your
Data Solutions?


Wide Industrial Coverage

Security and Confidentiality

Dataset Customization

Exceptional Workflow

Affordable Quick Services
We're here to help with
any questions
Get In touch
Maximise Potential with Macgence’s
Data Generation and Collection Services
powering AI projects and driving innovation.